According to a study by the European Commission, the fashion industry is responsible for two to ten percent of environmental pollution. In particular, the waste of unsold goods, which accounts for four percent of the worldwide waste produced annually, is a major challenge.
It is time to counteract this problem. Precise demand forecasts, for example, would enable companies to meet the demand for their goods without excessive inventory levels. They would also avoid the associated costs of overproduction, storage and disposal.
In addition, more precise requirements planning would have a positive impact on the environmental damage since precise safety stock forecasts for products and the associated “clothing waste” could be reduced.
Kerstin Schümann, student of eCommerce at the University of Applied Sciences in Wedel (FH Wedel), has dealt with the topic in her final thesis. In cooperation with minubo she examines the challenges of demand forecasts in retail and which approaches could be used in the future to optimize this process.
The Challenges in Retail
For many companies in the fashion industry it is a challenge to forecast the demand for their goods. Fashion companies often have a large product range with a large number of product variations, and the change in the fashion industry to the “fast fashion” trend also makes it difficult to forecast demand. Due to frequent product range changes and the short product lifecycles, there are only short sales histories.
This makes it increasingly difficult to predict sales figures and results in large quantities of clothing, which are often left unsold on the shelves. In addition, external factors such as fashion trends, competition and weather have a major impact on demand and can lead to overproduction.
If the demand is overestimated, companies not only have to expect excessive storage and administration costs, they also have a lot of tied-up capital that cannot be used for other purposes. If demand is underestimated instead, delivery bottlenecks can occur, which means that customer inquiries cannot be served. This increases the opportunity cost of a company because it makes less profit than would have been possible.
It is only through precise demand forecasts that companies can estimate the resources required for a planning period and exploit their full potential.
Demand forecasting in the fashion industry is a complicated and time-consuming task. As with the scientific work by Kerstin Schümann, many research projects deal with data science technologies to optimize and simplify the demand forecasting process and thus generate a data-driven basis for business decisions.
Machine Learning for More Accurate Forecasts
This could be remedied by technologies such as machine learning. Because the development of growing data volumes, in particular, creates the possibility to use this data for information generation and decision making.
Intelligent algorithms can support demand forecasting and thus, in addition to determining future demand, can also contribute to company-relevant planning calculations such as sales planning, procurement, production and financial planning.
Methodic Procedure
In the research project of Kerstin Schümann, two suitable machine learning algorithms were identified, presented and compared with each other. The objective was to find suitable algorithms for the forecasts, as well as a suitable forecasting method that meets the requirements of the fashion industry and delivers valid results.
For the study, the DeepAR forecasting algorithm was chosen, which was developed by Amazon for the creation of forecasts. This is based on recurrent neural networks, that is, the imitation of the human brain. Second, the SARIMA algorithm was found to be relevant, a classic machine learning approach to predict time series.
Both algorithms were set up and tested on the same data basis from a minubo customer. To generate a sales history of 2 years as the basis for the forecasts, product groups were created on the basis of product characteristics. A total of 20 groups were tested in order to be able to make a general statement about the forecasting ability of the algorithms. A distinction was made between product groups with high sales figures and product groups with average sales figures.
Conclusion
Basically, the analysis showed that a demand forecast can be made using machine learning algorithms and that the applied algorithms can efficiently generate forecasts for a large number of products. However, the results of the 20 categories examined showed that both algorithms have a tendency to underestimate the sales figures (positive difference).
The prerequisite for the successful use of these technologies is, among other things, the availability and quality of the database. The algorithms used can only "learn" and thus deliver better results in the form of meaningful analyses and recommendations for action if they obtain findings from the most comprehensive, up-to-date and high-quality data possible
It can also be stated that for the forecast with the most frequently sold categories, the SARIMA algorithm with a difference of approximately 12 percent has a significantly smaller deviation than the DeepAR algorithm with a difference of 64 percent. This is believed to be related to the “learning behavior” of the respective algorithm.
The study carried out is regarded as the basis for further analyses. In order to improve precision in the future, further product features can be implemented and tested in the algorithms.
This blog post is based on the results of the research project "Demand Forecasting through Machine Learning in the Fashion Industry" by Kerstin Schümann. She continues to work as a working student at minubo. If you have detailed questions about the study, please send us an email.
If you want to be informed about news in the future, subscribe to our monthly Commerce Insights newsletter.
About FH Wedel: The Fachhochschule Wedel University of Applied Sciences, located in the Hamburg metropolitan area, has stood for interdisciplinary, practical and international studies for more than 70 years. The university values the combination of practice and theory. To give students the opportunity to solve real problems and industry challenges, the Fachhochschule cooperates with about 200 partners from industry and economics – and minubo has been a partner since 2017.
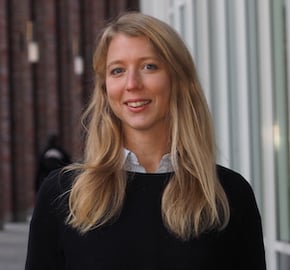